Sensor Based Adaptive Learning - Lessons LearnedAlbrecht Fortenbacher, Manuel Ninaus, Haeseon Yun, René Helbig, Korbinian Moeller
|
![]() |

Zusammenfassungen
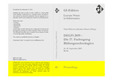
Dieses Konferenz-Paper erwähnt ...
![]() Begriffe KB IB clear | Bildungeducation (Bildung)
, ![]() ![]() ![]() ![]() ![]() ![]() |
Dieses Konferenz-Paper erwähnt vermutlich nicht ... 
![]() Nicht erwähnte Begriffe | Digitalisierung, LehrerIn, Schule, Unterricht |
Tagcloud
4 Vorträge von Beat mit Bezug
Zitationsgraph
Zitationsgraph (Beta-Test mit vis.js)
2 Erwähnungen 
- Jahrbuch Medienpädagogik 17 - Lernen mit und über Medien in einer digitalen Welt (Klaus Rummler, Ilka Koppel, Sandra Aßmann, Patrick Bettinger, Karsten D. Wolf) (2020)
- Lehren lernen mit digitalen Medien (Falk Scheidig)
- Lehren lernen mit digitalen Medien (Falk Scheidig)
- Informatik - Bildung von Lehrkräften in allen Phasen - 19. GI-Fachtagung Informatik und Schule (Ludger Humbert) (2021)
Anderswo finden
Volltext dieses Dokuments
Anderswo suchen 
Beat und dieses Konferenz-Paper
Beat hat Dieses Konferenz-Paper während seiner Zeit am Institut für Medien und Schule (IMS) ins Biblionetz aufgenommen. Beat besitzt kein physisches, aber ein digitales Exemplar. (das er aber aus Urheberrechtsgründen nicht einfach weitergeben darf). Aufgrund der wenigen Einträge im Biblionetz scheint er es nicht wirklich gelesen zu haben. Es gibt bisher auch nur wenige Objekte im Biblionetz, die dieses Werk zitieren. Beat hat Dieses Konferenz-Paper auch schon in Vorträgen erwähnt.